Tutorial
on Object Representation |
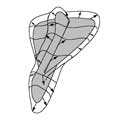 |
Much
of our work on segmentation is based on medial
geometry and the statistics of various object representations
|
|
|
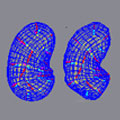 |
Object
representations provide a basis for segmentation by deformable models.
We have segmentation approaches by deforming
m-reps , deforming spherical harmonics representations, and deforming
dense positional grids using diffeomorphic fluid deformations. We
can also simulate intra-patient deformations via multigrid finite
element models using m-rep based meshing. |
|
|
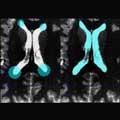 |
We
also work on segmentation via geometric flows. A general tool is provided
by SNAP.
Geometric
flows (PowerPoint file) together with statistical pattern recognition
is applied for the segmentation of brain tumors |
|
|
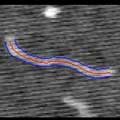 |
Medial
representations are used not only for segmentation by deformable object
models but also by direct medial analysis of image data. The resulting
loci are called "cores"
(PowerPoint file). We have a project on core extraction and have developed
a method of locating image objects via their medial features using
core
atom statistics. |
|
|
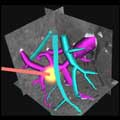 |
Segmentation,
registration, visualization, tracking, characterization, and quantification
of tubular
networks with an emphasis on networks of blood vessel for disease
detection and diagnosis and surgical planning, guidance, and outcome
assessment has also been a large
area of our work. |
|
|
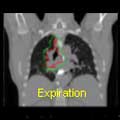 |
We
are beginning to be concerned not only of segmentation within single
images but also with spatiotemporal segmentation , i.e., segmentation
within time series of images. |
|
|
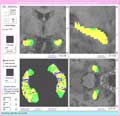 |
Segmentation
methods must be verified
(PowerPoint file). Part of our research focuses on the development
of methodology for the verification of segmentation. |
|
|
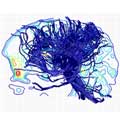 |
Lately
we have begun development efforts concerning the processing and visualization
of magnetic resonance diffusion tensor imaging (DTI) applied to brain
fiber tract analysis. |